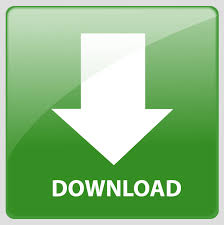
- #Asreml github how to#
- #Asreml github pdf#
- #Asreml github trial#
dropsVarComp Fitted model formula #> grain.yield ~ scenarioFull + scenarioFull:trial + (1 | genotype) + (1 | genotype:scenarioFull) #> #> Sources of variation #> component % variance expl. # Fit a model where trials are nested within scenarios.
As a mixed model, with the bold terms as random and the others as fixed. This can be specified using the engine parameter.įor diagnostic purposes and to identify the main sources of variation, all models are fitted three times: Be aware that when plot data are provided as input data, the mixed model analysis will be based on the assumption of completely randomized trials, which in almost all cases will not be appropriate for multi-environment trials.Īll models can be fitted using either lme4 or asreml. If the G圎 means are provided together with weights, then a residual term will be added to the models above. Trait = scenario + scenario:trial + genotype + genotype:scenario + genotype:scenario:trialįor data in the form of G圎 means, the last random term in all models above will become a residual term. Trait = region + region:location + year + region:year + region:location:year + genotype + genotype:region + genotype:region:location + genotype:year + genotype:region:year + genotype:region:location:year Trials correspond to locations within regions across years Trait = location + location:trial + genotype + genotype:location + genotype:location:trial Trait = year + year:trial + genotype + genotype:year + genotype:year:trial Trait = year + location + year:location + genotype + genotype:year + genotype:location + genotype:year:location Trials form a factorial structure of locations x years #Asreml github trial#
Trait = trial + genotype + genotype:trial These models are described in the table below, together with the function parameters used in gxeVarComp to fit the model. Six different types of models can be fitted depending on the structure of the environments in the data. In the statgenG圎 package this can be done using the gxeVarComp function. To investigate the structure of the genotype by environment data various mixed models can be fitted. Mixed model analysis of G圎 table of means In practice precision of the output can always be specified by the user. Note that due to technical restrictions the number of significant digits printed in tables throughout this vignette is not always optimal.
#Asreml github pdf#
Modeling of heterogeneity of genetic variances and correlationsįor most of the analyses a pdf report can be created automatically, see Reporting. Mixed model analysis of G圎 table of means. The following types of analysis can be done using statgenG圎: Further suggested reading is van Eeuwijk, Bustos-Korts, and Malosetti (2016). The availability of functions in the package is based on the analyses described in Malosetti, Ribaut, and van Eeuwijk (2013). #Asreml github how to#
This vignette describes how to perform the different types of analysis that are available in the package. The statgenG圎 package is developed as an easy-to-use package for Genotype by Environment (G圎) analysis for data of plant breeding experiments with many options for plotting and reporting the results of the analyses.